Learn About Propensity Score Matching At The Nashville Analytics Summit
We’re proud to announce that our lead data scientist,Michael Faron, will be presenting at the Nashville Analytics Summit.
TheNashville Analytics Summitwas created by Nashville Technology Council members and continues to expand as one of Nashville’s largest, locally grown tech events in the region. It focuses on fundamental issues in organizations today: how to effectively leverage big data and analytics.
Michael will be talking about how organizations can leverage Propsensity Score Matching (PSM) to measure the effectiveness of analytics technology.
Michael is not only super intelligent, but he’s funny, engaging and has the hard-to-find ability to uncomplicate complicated topics. If you’re planning to attend the Summit, make sure to check out our guy, Michael.
Here’s Michael’s quick summary of what PSM is and how it’s been particularly effective when conducting healthcare studies:
At Medalogix, our Touch and Bridge products leverage predictive analytics and clinical workflows to help clinicians manage their patient population in the Home Health and Hospice settings. The goal is to improve outcomes, but how can we know if the tools and processes are effective? One of my responsibilities as director of data science at Medalogix is to evaluate the validity of our solutions.
When we examine the data our customers and tools are collecting over time, we expect to find differences in outcomes for patients who receive care under Touch vs. those that do not. But when differences are found the first thing that comes to my mind is the statistics mantra that “correlation does not imply causation.”
In clinical research settings, randomized controlled trials (RCT) are an indispensible approach to understanding and demonstrating causation and the effectiveness of a treatment, tool or process. However, RCTs can be expensive, time consuming, and in many cases impractical or even unethical. But when data is available, yet it did not come from an RCT, there is still a way to move forward on understanding a true treatment effect.
Propensity Score Matching (PSM) is an analytics technique that delivers the benefits of an RCT without having to do one. With PSM, two things are needed. First, the patient data you have must be divisible into treatment and control groups. In my case this split was defined as patient exposure to Touch vs. no exposure to Touch. Second, additional patient variables commonly available from an EMR such as gender, age, cancer risk, etc. are needed. PSM use these additional variables to take an apples-to-oranges situation to an apples-to-apples situation.
Let’s just take a brief moment to peek at how this is done. With RCTs, subjects in the control and treatment groups are theoretically similar in all characteristics, both observed and unobserved because of random assignment. There is no inherent that one group would be older than the other, or one group would have a greater risk of cancer, and so on. RCT is apples-to-apples, except for the treatment.
But when using EMR data, or “observational data,” there is selection bias. For example, at Medalogix we often find that patients associated with Touch and Bridge are higher-risk patients. This makes sense in a resource-constrained world where tools and need often coincide under clinician care. Thus, when looking at patients who did and did not receive treatment, we have an apples-to-oranges comparison. Therefore, any outcome difference could be due to patient differences, not simply the care choice of Medalogix Touch. PSM identifies similar patients in aggregate across the two groups by assigning each subject a propensity score via the additional balancing variables. Those with similar propensity scores are considered equally like to have been in the treatment or control group. In a sense, randomization has been “reverse-engineered” into the data to overcome selection bias.
In a recent study at Medalogix for our Touch product, I used PSM to analyze data from 1,426 patients receiving Touch vs. 6,687 control patients. It was immediately clear that an apples-to-oranges situation existed in the data. Touch patients scored higher on our predictive model in regards to transfer risk, and there was clear group imbalance across patient covariates such as age, medication use, ambulatory status, diagnosis risk and other risk-related variables. With PSM, I was able to account for selection bias and create an apples-to-apples comparison to estimate a treatment effect for Medalogix Touch, and the results are in.
We found that the patients receiving care under Medalogix Touch had a significantly lower risk of transfer off census.The absolute risk reduction (ARR) was 5.5%.
Even though randomized controlled trials are the preferred choice for understanding treatment effects, they are not always feasible or possible. Propensity score matching is a well-established analytics technique that can estimate treatment affects using everyday, non-experimental data by changing an apples-to-oranges situation into an apples-to-apples comparison.
Related Blogs
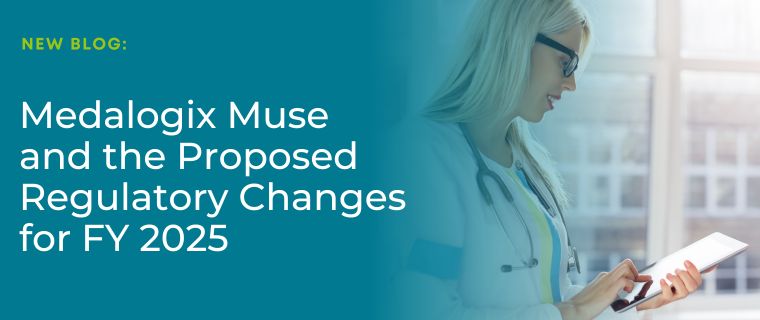
Medalogix Muse and the Proposed Regulatory Changes for FY 2025
Steven Shelton, MBA, MSN, RN, CHPN; Director, Clinical Services In th...
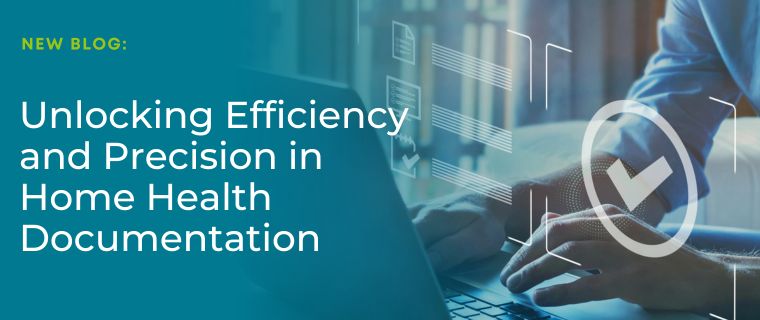
Unlocking Efficiency and Precision in Home Health Documentation
Home health teams operate in diverse and uncontrolled settings, ranging ...
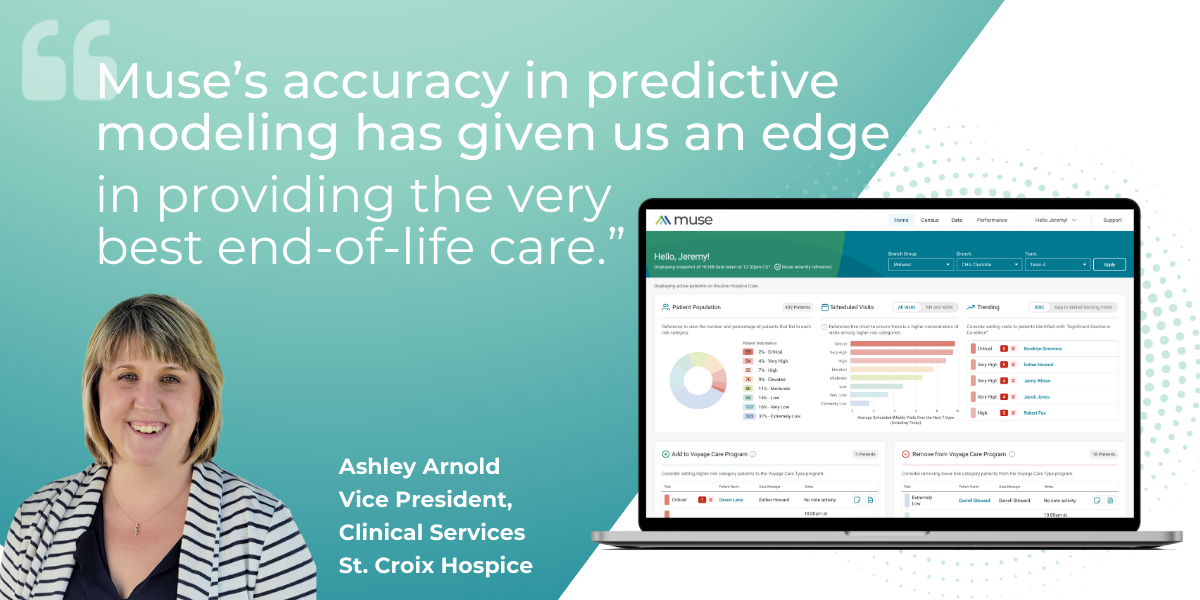
Transforming End-of-Life Care with Medalogix Muse at St. Croix Hospice
St. Croix Hospice, a nationally acclaimed hospice agency, has leveraged ...