Medalogix Helps Signifigantly Reduce Avoidable Hospital Readmissions. Our Data Scientist Can Prove It.
Our lead data scientist has analyzed the effectiveness of our readmission reduction solution solution and the results are in. Drum roll please….
We’ve helped home health clients reduce their avoidable readmissions by27-37%!In addition to reducing readmission rates, home health agencies leveraging Touch have also found success using the technology to help manage patients in bundles or value-based purchasing states.
Touch offers cascading benefits also. For instance:
- It’s built in automated calling functionaugments in-person home health visits with IVR or scheduled manual calls. This helps improve patients care.
- Helps improve patient satisfaction. More touchpoints via automated calling typically helps patients feel more at ease because they know they’re being checked on in between visits.
- By predictingand ranking which patients are most at risk for readmission, you can help ensure busy cliniciansare dedicating their attention where it’s most needed at that specific time.
Medalogix data scientist’s havereproduced our Touch readmission reduction study at 5 different home health agencies, repeatedly finding statistically significant results. We’ve even had Vanderbilt data scientists peer review our work. Here’s a chart that highlights those agencies’ absolute and relative readmission reduction rates.
Here’s some information from our lead data scientistabout propensity score matching (PSM) –the testingtechnique he used to study the effectiveness of our Touch technology:
Purpose
The goal of the study is to estimate the effect of MedalogixTouchapplication at a home health agency (HHA) on reducing their patients’ transfer to hospital, skilled nursing facilities or hospice. It is an observational study in which patient and outcome data are collected retrospectively. An immediate challenge is the selection bias due to the nonrandomized nature of the observational study. The study uses propensity score matching to address such potential treatment selection bias and from which to estimate the genuine average effect of the treatment for the treated (ATT).
Methodology
Propensity score one-to-one matching method is adopted. First, the Medalogix research team estimated a logistic regression model in which the treatment status (under Medalogix Touch care) is regressed on patient’s observed baseline characteristics. Second, patients are scored by the model.One-to-one score matching is implemented to select control patients who are the closest to the treated patients with respect to the logit of their propensity scores. The threshold difference is 0.20 of a standard deviation of the logit of the propensity scores.After matching, the analysis on outcome provides a valid estimate of treatment effect because the research method compares patients with matched observed characteristics, all of whom are potential candidates for the treatment.
Model Validation and Robustness
The Medalogix research team uses the re-sampling technique (bootstrapping 3,000 times) on matched pairs to test for the robustness of estimates. Bootstrapping has the advantage of distribution-free assumptions on estimate testing.
Interested in learning more about Touch’s effectiveness? check out thiscase studyon Medalogix Touch.
Interested in learn more about why you should be using PSM for ROI so you are always comparing apples-to-apples. Here’s ablogon that topic from our head data scientist, Michael Faron.
Related Blogs
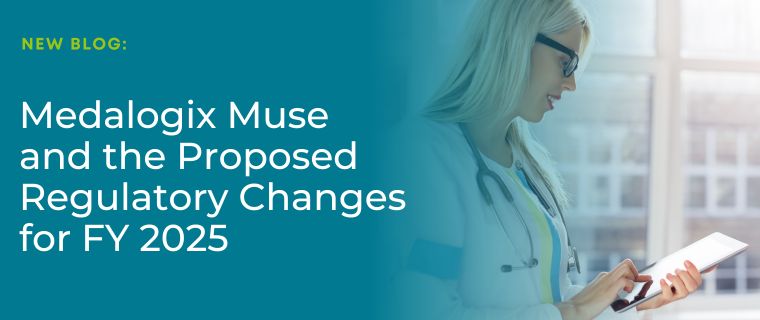
Medalogix Muse and the Proposed Regulatory Changes for FY 2025
Steven Shelton, MBA, MSN, RN, CHPN; Director, Clinical Services In th...
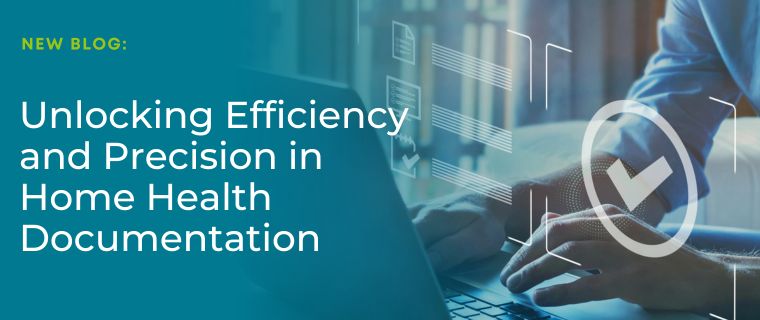
Unlocking Efficiency and Precision in Home Health Documentation
Home health teams operate in diverse and uncontrolled settings, ranging ...
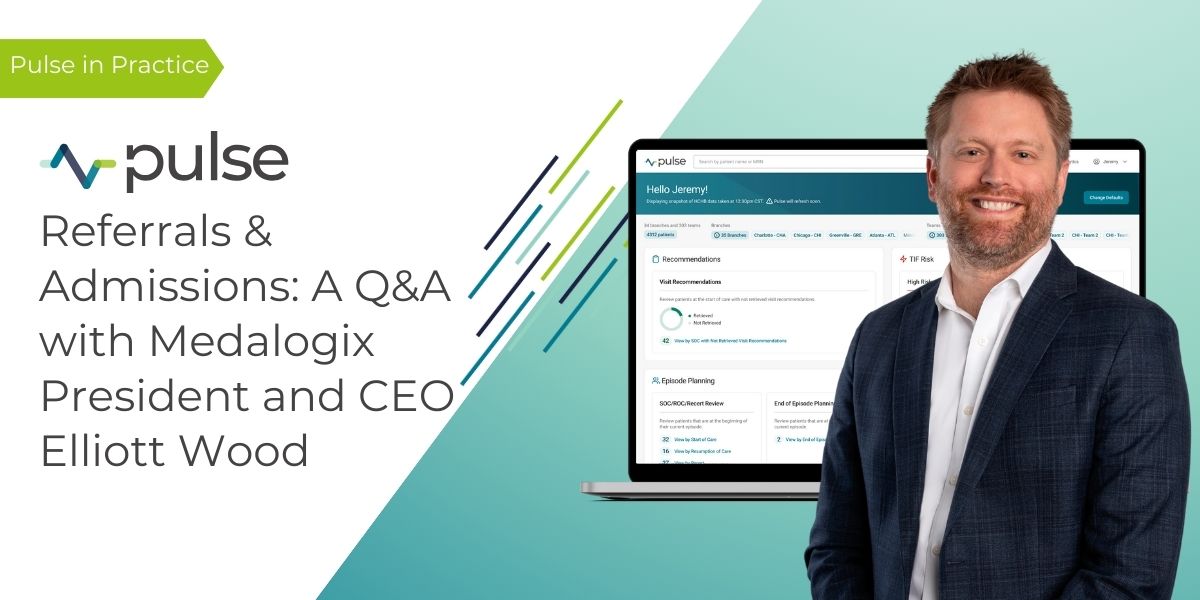
Pulse Referrals & Admissions: A Q&A with Medalogix President and CEO Elliott Wood
With our new Referrals & Admissions module now available for purchas...