How To Assess Readmission Reduction Technologies In Preparation for Home Health VBP
Have questions about the best way to navigate Home Health Value Based Purchasing? We’ve got answers.
With the recent introduction of the Home Health Value Based Purchasing program, CMS has emphasized the important role home health will play in reducing not just unnecessary readmissions, but unnecessary admissions within the continuum of care.
Home Health companies will have to begin evaluating cost-effective strategies for preventing unnecessary hospital admissions. Identifying strategies and programs that actually prevent unnecessary admissions is an extremely difficult task (hence the focus CMS is forcing onto the industry). Additionally, we’vefound that effectively measuring the impact ofa good strategy isequally, if not more challenging.
Here is an measurement component that we have used to measure the effectiveness of readmission reduction technologies. I’d like to geek out on data science for a minute and share it with you–don’t worry, I’ll steer clear of too much nerd talk:
Propensity score matching 101:
When you begin to design your measurement approach regarding a specific intervention with your patients, like a readmission reduction solution, a key design element you have to get right is the part about comparison. The difficult question is:
How will you compare the patients whoreceive the readmission reduction treatment/intervention you have implemented vs. those that have not?
We found that it is usually NOT good enough to look at readmission rates over time or readmissions in different branch locations, even though this is the easy way out. The challenge here is acuity. How do we know that the populations we are measuring against each other are the same? They need to be the same in order to determine if the treatment you have selected is actually causing an impact!
This is why researchers love randomized controlled trials. Let me walk you through a silly example:
There is a researcher who has developed a new pill to reduce heartburn after holiday meals (last week were the holidays, so good food is on my mind). She has finalized the scale for assessing heartburn levels after the meal, and she wants to see if the pill will actually reduce levels of heartburn after dinner with her family.
A good researcher will use randomization to ensure the population who takes the pill looks very similar to the population that does NOT take the pill. She would then let everyone eat holiday dinner, and measure the difference between the family members that took the pill and those who did not.
If she just wanted to cheat the system and say her pill worked, she could simply give the pill to all of the family members under 35, and show a lower heartburn experience after dinner than the parents and grandparents at the party.
This is a silly example that is meant to be illustrative. The point is, randomization reduces the bias associated with selecting groups, which gives the researcher the best ability to make sure the treatment group (the family members that got the pill) and the control group (those who did not get the pill or had a placebo) are as similar as possible, so that she can see if the pill is actually what makes the difference.
The problem is, home health providers who are being subjected to reimbursement penalties for readmission don’t have time to set up randomized control trials for every single patient intervention program they want to deploy.Home Health providers (in 9 states) were told by CMS in mid-2015 that HHVBP was happening, and they would effectively be “on the clock” starting 1/1/2016.
Therefore, how do you compare your treatment programs effectively and efficiently? We have started using a technique called propensity score matching. Any vendor you’reworking with to reduce readmissions (telehealth, convener services, etc…) who claims to have a predictive model or a risk flag for readmission should be able to do this for you.
In the case of unnecessary admission reduction, propensity score matching works by using a predictive model to match patients that received the treatment with those that did not.
We have leveraged this technique multiple times with clients using MedalogixTouch, our readmission reduction solution. Touch helps home health providersincrease Touchpoints, either manually or with our Integrated Voice Response (IVR) system. Our clients want to know if their efforts leveraging Touch are working.
Here is a graph which compares several variables we used to determine the patient’s likelihood (propensity) to readmit in both the treatment group (those who received calls) and the control group (those who did not).
Before Propensity Score Matching
Ignore all of the fancy numbers on the vertical axis. Do you see the dots? Those are a few variables within our model, contributing to the patient’s likihood to transfer to the hospital from home health (i.e. # of Medications, Ambulation and Transfer Risk, etc…) Do you see the two dotted lines across the chart? That is a measurement of the two groups (treatment and control), and how similar these groups actually are. The fact that you see dots outside of the two dotted lines means the groups we are wanting to compare are not actually comparable. They have different acuity levels!
Therefore, it is not good enough to simply look at the patients they were called vs the patients they were not called. Why? Because one group is actually just sicker, and a difference in their admission rates may not have anything to do with our solution or the client’s clinical program.
Here is a chart that shows the comparison of these two groups after propensity score matching:
After Propensity Score Matching
Now, we have matched the patients with similar acuity levels and can review their outcomes (i.e. TIF rates within 60 days) to make an informed decision about the efficacy of the program. There is obviously more to setting up the process like standardizing the results, ensuring you have a large enough N that you can determine if the findings are significant, and more. But this is an efficient technique you can leverage to begin assessing the effectiveness of your programs, which will become more and more critical as we move further into the era of quality reform.
The next step in the process should be converting your findings into a relevant measure that allows you to measure the value of your program to your organization. On example metric is the “number needed to treat” (NNT).With one client, we found for every 14 patients they called with Medalogix Touch and the patient confirmed a clinical problem, they were able to prevent a transfer to the hospital (that they would have otherwise missed had the program not been in place).In a value based purchasing world, these prevented TIFs will be very valuable.
*Photo Credit:quickmeme.com
Related Blogs
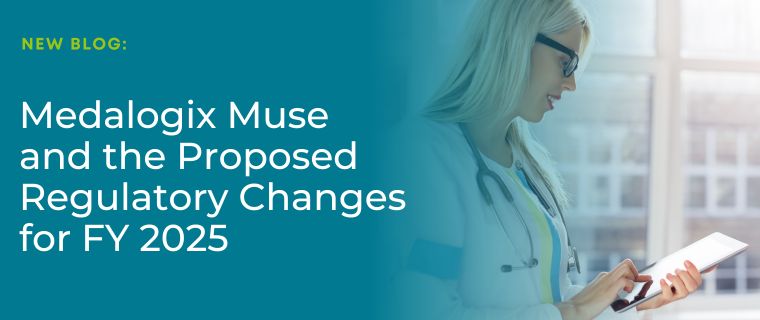
Medalogix Muse and the Proposed Regulatory Changes for FY 2025
Steven Shelton, MBA, MSN, RN, CHPN; Director, Clinical Services In th...
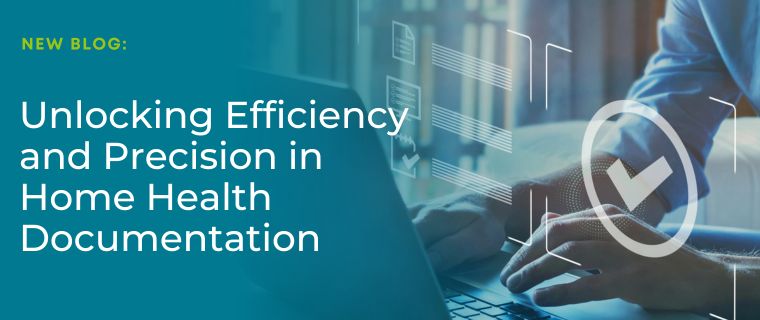
Unlocking Efficiency and Precision in Home Health Documentation
Home health teams operate in diverse and uncontrolled settings, ranging ...
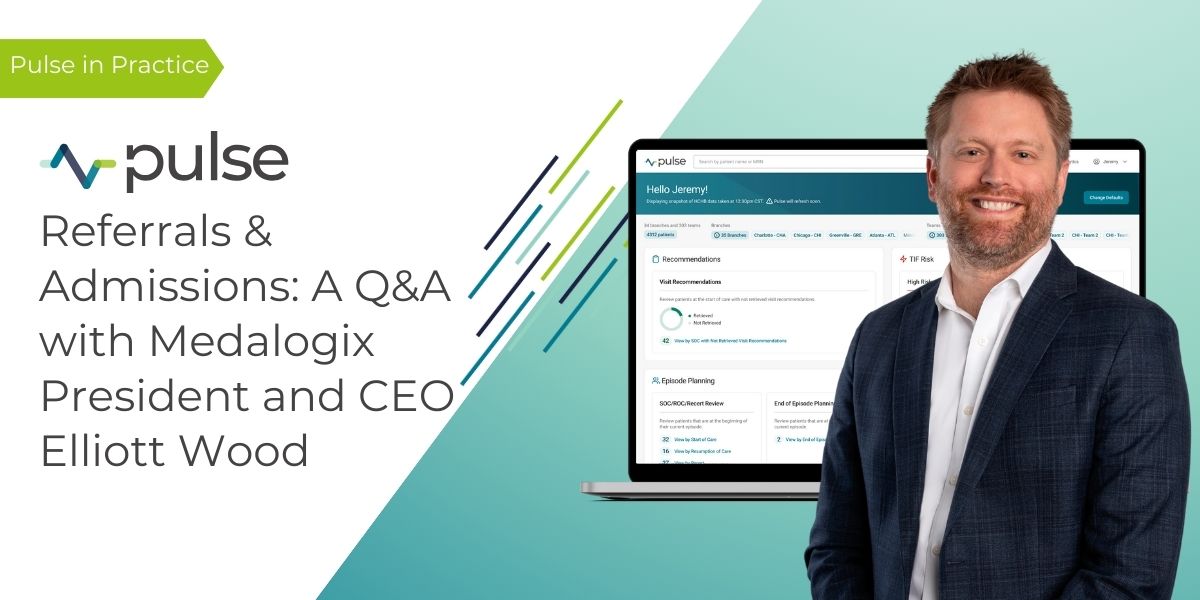
Pulse Referrals & Admissions: A Q&A with Medalogix President and CEO Elliott Wood
With our new Referrals & Admissions module now available for purchas...